82 MACHINE LEARNING APPLIED TO TOURISM – Contributions by Célia M.Q. Ramos
1. Introduction
Due to its economic and regional importance, tourist activity is a strategic sector of economic and social development, even apart from considering its environmental component. In this perspective (Ramos et al., 2020), it constitutes a privileged field of investigation, essential to the understanding and analysis of the various phenomena that underlie it and that, in most situations, support the aspects of differentiation that motivate the international competitiveness of destinations, while helping their sustainability (Ramos et al., 2020; Cardoso et al., 2020a).
For Sheldon (1989, p. 589), “information is the lifeblood of the tourist industry”; travellers, travel agencies, suppliers and all stakeholders in the tourist distribution chain need information. Optimizing information and communication technologies (ICT) has allowed tourist organizations to improve the flow of information, improve response times to requests and increase their productivity in such a way that organizations started to have similar technological bases to guarantee their survival (Ramos et al., 2015).
In recent decades, innovative technologies have affected tourism activity (Ramos & Rodrigues, 2013), with each new technology quickly adopted after its development. ICT is used in all travel phases, from the beginning, during and after the trip, to find information, book products, share personal histories and create digital memories.
During the travel phases, ICT is the main driver of the personalization of the tourism experience (Buhalis & Amaranggana, 2015). Before the trip, it can help the traveller to obtain information about how to access transport, hotels, and/or local gastronomy; during can contribute to access in real-time to personalized services and information. After can be used to generate testimonial content, receive promotions, or share experiences with other tourists (Antunes et al., 2018).
Additionally, technology potentiates the development of smart tourism destinations by facilitating the collection of insights into customers’ actual needs and preferences (Buhalis & Amaranggana, 2015) in an environment where personalization of the experience is the most crucial objective.
In smart destinations, technology creates bridges between digital and physical through integrating sensors and smartphones (Rodrigues et al., 2016), where big data has become more relevant than simple information during travel. The challenge in this ecosystem is not interactivity but co-creation through ICT (Gretzel et al., 2015). For example, the traveller shares their testimony on social media that will be analysed by destination agents and travel agencies.
Today it is no longer enough to have a technology base to meet traveller needs (Ramos & Brito, 2020). The digital economy requires that tourism companies continuously adapt, especially to the digital transformation of their activity, with the emergence of a new paradigm associated with the theory of the fourth industrial revolution (Industry 4.0). Where it is necessary to consider advanced technological resources, such as information security in the virtual space; augmented reality; big data; cloud computing; the Internet of things (IoT); autonomous robots; 3D printing; integrated systems; and mobile Internet (Ramos & Brito, 2020).
2. Digital Transformation
Digital transformation is a new paradigm that contributes to the acceleration of business in tourism activities management and in all economic sectors (Ramos, 2022) to identify an adequate system to manage, store, connect, communicate, and automate information and where the travel experience should be the focus and the concept is customer-centric (Ramos, 2022).
The digital transformation associated with the tourism sector, as presented in Figure 1, where the activities are complementary, has changed the interaction between the suppliers and demand, affecting the customer’s journey and the decision-making process (Cuomo et al., 2021).
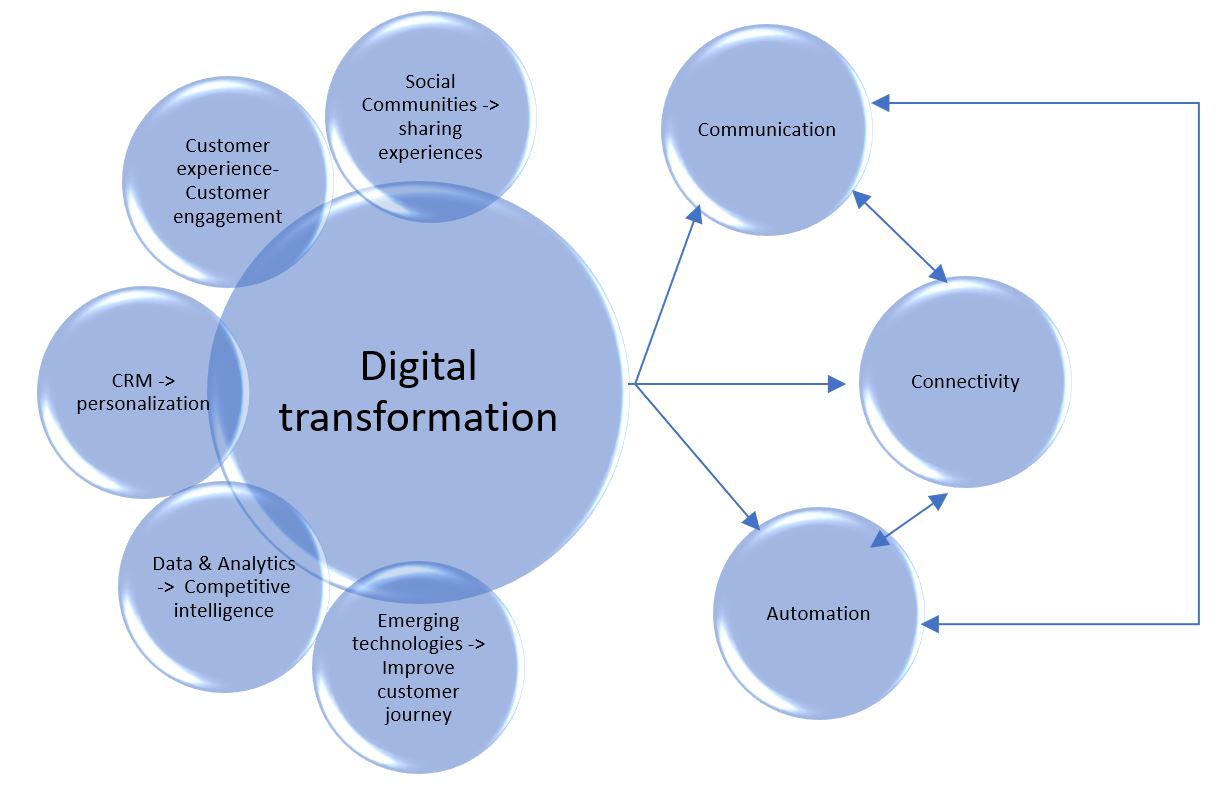
Digital transformation should allow the development of an environment that establishes a communication bridge in the digital medium between the company, consumers, and other consumers; should have excellent connectivity, with access from anywhere at any time and from multiple devices; and with process automation, so that responses and sales processing are carried out as quickly as possible, in any of the steps of the consumer decision-making process.
The consumer decision process is divided into five steps (Lemon & Verhoef, 2016): (a) recognition of a need or want; (b) search of product-related information to identify options; (c) analysis and evaluation of alternatives based on developed evaluation criteria; (d) product selection and action of purchase; and (e) consumer behaviour after the purchase, including product use, post-purchase services, service quality, satisfaction, word-of-mouth, and repurchase.
The traveller uses technology in all the steps of the decision-buying process. All the information searched on websites, and social media can be collected to personalize offers according to consumer preferences, but it also is a necessary tool for businesses to achieve insights related to customer behaviour and to create new opportunities (Ramos & Rodrigues, 2019; 2020; 2021). In this case, knowledge management and business intelligence can make a difference in support of tourism companies’ strategies.
Knowledge management is an area that can improve the information available for decision-making. It includes a set of intangible assets or competencies fundamental to obtaining a competitive advantage for the tourist company. Under the right conditions, it can improve the decision-making process, increase the organization’s ability to solve problems and increase its capacity to innovate (Ramos, 2022) by offering services and products according to customer preferences.
Knowledge management also contributes to increasing the competitive advantage of tourism organizations. It should be monitored and evaluated by intellectual capital indicators: relational, structural, or human capital, which can be used as key performance indicators for business intelligence tools (Ramos et al., 2017; Ramos & Perna, 2009).
3. Business Intelligence and Machine Learning in Tourism
Business intelligence systems combine data with analytical tools to provide information relevant to decision-making, intending to improve the quality and availability of this information, presenting valuable data for organizations in dashboards or reports with insightful information associated with the tourism business.
Combining intellectual capital indicators management concepts, business intelligence tools, and machine learning algorithms can contribute to knowledge creation and service innovation in tourism (Ramos et al., 2019; Ramos et al., 2017b).
The most competitive tourist organizations make strategic investments in artificial intelligence and machine learning (ML) to enable the automation of processes, make predictions, discover patterns in data, and discover rules. This can help the organization achieve business advantages, thus ensuring its competitiveness and survival in a digital economy with small profit margins.
Machine learning can be used to do tourism analyses which can be divided into four analytical areas: Descriptive: answer questions like “What happened?”; diagnostic: help answer the question “Why did it happen?”; predictive: respond to questions like “What will happen?”; and prescriptive: look at questions like “How can I make it happen again?”. For a tourism company:
- Descriptive analytics can be used to discover patterns associated with the tourism activity, behaviour or preferences, and customer segmentation (cluster segmentation).
- Diagnostic analytics can be used to understand causes and tourist travellers’ behaviours, for example, to identify rules that explain why tourists select one product instead of another (decision tree).
- Predictive analytics can deploy sets of statistical techniques to estimate models that predict future tourist visitors’ behaviours, for example, predicting customer ratings or the number of sales in a specific period (linear regression, logistic regression).
- Prescriptive analytics is used to optimize a key performance metric, for example, profit.
These combined business intelligence tools help the company to make responsive future-oriented decisions.
In the tourism economic sector, machine learning methods can be used in various ways. These methods can help to understand traveller sentiments, identify tourists’ characteristics, and predict the company’s reputation (Ramos & Casado-Molina, 2021; Ramos et al., 2017a). Also, it can use reviews to discover preferences and anticipate customers’ problems, discover what influences buying behaviour, identify potential competitive advantages, improve guest satisfaction, develop more effective loyalty programs, and obtain insights about brand reputation.
For example, in combining relational capital indicators with machine learning methods, it is possible to research customer loyalty by obtaining a 360-degree view of each customer, discover patterns and the causal relationship that contributes to customer satisfaction and loyalty, and develop predictive models that anticipate future earnings, learn from the customer interaction and behaviour to define business strategies and better understand tourism decision-making, identify what kind of services or products are commonly purchased together, improve sales forecasting, predict customer churn, identify fraud, measure performance, and define more efficient key performance indicators (Sharma & Sharma 2016).
Regarding the phases of the trip, machine learning can be used before the trip to forecast tourist demand as well as cancellations in hotels, transport, restaurants, and amusement parks. During travel, ML algorithms can be considered in recommendation systems, which can work by analysing traveller preferences. In the post-trip phase, these algorithms can be used to analyse the reviews produced on social media, with testimonies of the tourist experience (Afsahhosseini & Al-Mulla, 2020; Ramos & Hassan, 2021).
Machine learning is a branch of artificial intelligence that develops algorithms with the ability to learn without being explicitly programmed (Alzubi et al. 2018; Mitchel, 1997). There are different types of learning, among them, supervised and unsupervised learning. The first involves algorithms to learn from previously labelled data. The learning process will classify the data based on their relationships in the second type, where the data are not already labelled. An appropriate ML method should be applied depending on the problem and variable types. This can include the following methods (Egger, 2022):
- Logistic regression – Supervised learning with a Boolean-dependent variable can be applied to the definition of a model that will allow estimating the reservation cancellation forecast.
- Linear regression – Supervised learning with a continuous dependent variable can be applied to define a model that will allow estimating the forecast of tourist demand.
- Decision tree – Supervised learning with a categorical variable can be considered an algorithm that combines classification with regression and can be applied to predict the evaluation attributed to the tourist experience.
- Classification – Supervised learning with a categorical dependent variable can be applied to divide tourists into different types of consumers.
- Segmentation or clustering – Unsupervised learning applied to continuous variables can be applied to segment customers, as it divides data into groups based on similar properties.
- Dimension reduction – Unsupervised learning applied to continuous variables can help find the best combination of variables that represent the preferences of each segment.
- Association rules — Unsupervised learning can be applied to identify products and/or services that the guests jointly purchase.
In addition to these algorithms, there is more to machine learning (Casado-Molina et al., 2019). In addition to numerical, continuous, or categorical data, there are other types, such as text, image, video, and voice. Text mining is increasingly a tool to be considered by the entities that govern tourist destinations to define communication, management, and monitoring strategies.
Social media play a relevant role in the sustainable development of tourism activity (Ramos & Hassan, 2021) through the analysis of the testimonies about traveller experiences expressed in this medium. Sentiments expressed about authenticity, destination identity (Marine-Roig, 2015), and sensorial experiences (Agapito, 2020; Cardoso et al., 2020b, Rodrigues et al., 2019) experienced during the trip are significant for the management of destinations to understand how to increase consumer satisfaction and to increase their loyalty, through the possibility of predicting tourists’ feelings (Ramos, 2022).
ML algorithms can be applied to social media reviews and help with sentiment analysis (SA), the field of study that analyses text for people’s opinions, feelings, evaluations, and attitudes.
Sentiment analysis can contribute to identifying the leading influencers of tourist behaviour in the decision process, which is motivated by consumer beliefs, perceptions, and testimonies expressed in the digital environment. This method also helps businesses to understand the dimensions associated with the image of tourist destinations; the associated correspondent quality of service; the identification of tourists’ preferences in accommodation, catering, gastronomy, and transport; the culture of the destination (for example, museums); and the quality of life and well-being of both tourists and residents.
4. Future Research
Digital transformation will continue to take place, and despite its numerous benefits, it also has negative impacts. A new growth model should be adopted based on the concepts of Society 5.0. which includes data collected second by second from existing sensors in physical space, collected by IoT or drones.
These data will be stored in virtual space, creating a big data giant that will have to be analysed with artificial intelligence methods that communicate with humans in physical devices with different formats. For example, through exoskeletons, technology additive to print specific products, where medical information will help to have a longer, healthier and well-being life, whose data will be protected with Blockchain technology (Ramos, 2021) and communicated between systems through 5G technology.
In Society 5.0, the focus will be on the human being. At the same time, for companies, the main drivers are the customer experience, hyper-customization, automation of the distribution chain, and interaction between products to increase the experience and activate new functionalities, characteristics provided by the fifth technological revolution (industry 5.0). This revolution goes beyond producing goods and services for profit, placing worker well-being at the centre of the production process, integrating ethical considerations in developing intelligent interactive systems (Dignum, 2018), and considering research and innovation for a sustainable, human-centred, and resilient industry.
5. Conclusions
Technology has been a powerful ally of the tourism industry. The power of the relationship between companies and travellers has increased and, in parallel, has generated large dimensions of data that, with powerful analysis tools, present competitive advantages to destination managers. The trend is towards reaching the assumptions of a human-centred Society 5.0.
Digital transformation makes use of emerging technologies, which allow access to social communities in the digital environment with the use of tools to manage the relationship with the customer to involve the tourist consumer—the traveller—where data analysis allows the leverage of communication, automation, and connectivity between all those involved.
It will be increasingly important to use data analysis techniques based on artificial intelligence algorithms so that it is possible to place the consumer at the centre of the analysis to meet their preferences. Nevertheless, ethics and care for human beings have to go hand in hand with protecting their privacy. Only with this concern will it be possible to ensure a successful alliance between business, consumers, and technology, which will only be viable using business intelligence tools, machine learning algorithms, and human resources trained to work with these technologies.
Acknowledgements
This research has been financed by National Funds provided by FCT – Foundation for Science and Technology (Portugal) through project UIDB/04020/2020.
Written by Célia M.Q. Ramos, School for Management, Hospitality and Tourism (ESGHT) and Research Centre for Tourism, Sustainability, and Well-Being (CinTurs), Universidade do Algarve, Portugal.
Read Célia’s letter to future generations of tourism researchers
References
Afsahhosseini, F., & Al-Mulla, Y. (2020, September). Machine learning in tourism. In 2020 The 3rd International Conference on Machine Learning and Machine Intelligence (pp. 53-57). Doi: 10.1145/3426826.3426837
Agapito, D. (2020). The senses in tourism design: a bibliometric review. Annals of Tourism Research, 83, 102934.
Alzubi, J., Nayyar, A., & Kumar, A. (2018). Machine learning from theory to algorithms: an overview. Journal of physics: conference series, 1142(1), 012012.
Antunes, N., Ramos, C., & Sousa, C. (2018). Who are the Tourists Sharing Content on Social Media? Behaviour and Characteristics. Journal of Spatial and Organizational Dynamics, 6(3), 237-257.
Buhalis, D., & Amaranggana, A. (2015). Smart tourism destinations enhancing tourism experience through personalisation of services. In Information and communication technologies in tourism 2015 (pp. 377-389). Springer, Cham.
Cardoso, P.J.S., Cabrita, C., Cruz, D., Pinto, N., Monteiro, J., Semião, J., Ramos, C.M.Q., & Rodrigues, J.M.F. (2020a). Monitoring, Predicting, and Optimizing Energy Consumptions: A Goal Toward Global Sustainability. In Rodrigues, J.M.F., Cardoso, P. J.S., Monteiro, J., Ramos, C.M.Q. (2020). Smart Systems Design, Applications, and Challenges (pp. 80-107). Hershey, PA: IGI Global. Doi: 10.4018/978-1-7998-2112-0.ch005
Cardoso, P.J.S., Rodrigues, J.M.F., Pereira, J., Nogin, S., Lessa, J., Ramos, C.M.Q., Bajireanu, R., Gomes, M., & Bica, P. (2020b). Cultural Heritage Visits Supported on Visitors’ Preferences and Mobile Devices. Universal Access in the Information Society, 19, 499-513. Doi: 10.1007/s10209-019-00657-y
Casado-Molina, A.M., Ramos, C.M.Q., Rojas-de-Gracia, M.M., & Peláez-Sánchez, J.I. (2019). Reputational Intelligence: Innovating Brand Management Through Social Media Data. Industrial Management & Data Systems, 120(1), 40-56. Doi: 10.1108/IMDS-03-2019-0145
Cuomo, M.T., Tortora, D., Foroudi, P., Giordano, A., Festa, G., & Metallo, G. (2021). Digital transformation and tourist experience co-design: Big social data for planning cultural tourism. Technological Forecasting and Social Change, 162, 120345.
Dignum, V. (2018). Ethics in artificial intelligence: introduction to the special issue. Ethics and Information Technology, 20(1), 1-3.
Egger, R. (Ed.) (2022). Applied Data Science in Tourism: Interdisciplinary Approaches, Methodologies, and Applications. Springer Nature.
Lemon, K.N., & Verhoef, P.C. (2016). Understanding customer experience throughout the customer journey. Journal of Marketing, 80(6), 69-96.
Marine-Roig, E. (2015). Identity and authenticity in destination image construction. Anatolia, 26(4), 574-587.
Mitchell, T.M. (1997). Machine learning. Burr Ridge, IL: McGraw Hill. ISBN 978-0-07-042807-2.
Ramos C.M.Q., & Rodrigues J.M.F. (2019). The Contribution of Social Networks to the Technological Experience of Elderly Users. In: Antona M., Stephanidis C. (eds) Universal Access in Human-Computer Interaction. Multimodality and Assistive Environments. HCII 2019. LNCS 11573, pp. 538-555. Springer, Cham. Doi: 10.1007/978-3-030-23563-5_43 ISBN: 978-3-030-23562-8
Ramos C.M.Q., & Rodrigues J.M.F. (2020). The Influence of Social Networking Technology on Centennials Purchase Intent. In: Antona M., Stephanidis C. (eds) Universal Access in Human-Computer Interaction. Applications and Practice. HCII 2020. LNCS 12189, pp. 451–465. Springer, Cham. Doi: 10.1007/978-3-030-49108-6_32
Ramos C.M.Q., & Rodrigues J.M.F. (2021). Social Network Behavior, from Information Search to Purchase: The Case of Generation X and Millennials. In: Antona M., Stephanidis C. (eds) Universal Access in Human-Computer Interaction. Design Methods and User Experience. HCII 2021. Lecture Notes in Computer Science, vol 12768. Springer, Cham.
Ramos, C.M.Q., & Rodrigues, P.M.M (2013). Research note: The importance of online tourism demand. Tourism Economics, 19 (6), 1443-1447. Doi: 10.5367/te.2013.0253. ISSN: 1354-8166.
Ramos, C. M. (2021). Blockchain Technology in Tourism Management: Potentialities, Challenges, and Implications. In Blockchain Technology and Applications for Digital Marketing (pp. 84-109). IGI Global.
Ramos, C. M. (2022). Business Intelligence Approach and Sentiment Analysis as a Management Strategy Applied to Study Customer Satisfaction in the Hospitality Sector. In Advances in Tourism, Technology and Systems (pp. 537-547). Springer, Singapore.
Ramos, C.M., & Brito, I.S. (2020). The Effects of Industry 4.0 in Tourism and Hospitality and Future Trends in Portugal. In The Emerald Handbook of ICT in Tourism and Hospitality. Emerald Publishing Limited.
Ramos, C.M., & Hassan, A. (2021). Evaluation of the tourist experience using a framework for the analysis of the influence of social media on tourist behaviour. International Journal of Tourism Policy, 11(3), 207-221.
Ramos, C.M., Andraz, G., & Cardoso, I. (2020). The role of ICT in involving the tourist and in sustainability of tourism destinations. In Technological Progress, Inequality and Entrepreneurship (pp. 29-45). Springer, Cham.
Ramos, C.M., Rodrigues, P.M., & Rodrigues, J.M. (2015). Opportunities, emerging features, and trends in electronic distribution in tourism. International Journal of Information Systems and Social Change (IJISSC), 6(4), pp.17-32.
Ramos, C., & Perna, F. (2009). Information System for Tourism Activity Monitoring and Forecasting Indicators as an experience for Portugal. Tourism and Hospitality Research, 9 (4), 277-289. Doi:10.1057/thr.2008.45. ISSN: 1467-3584.
Ramos, C.M.Q., Casado-Molina, A.M., & Peláez, J.I. (2017a). Measuring air and terrestrial transport company reputation: tourism intangibles expressed in the digital environment. Journal of Spatial and Organizational Dynamics, 5(4), 443-462. ISSN: 2183-1912.
Ramos, C.M.Q., Martins, D.J., Serra, F., Lam, R., Cardoso, P.J.S., Correia, M.B., & Rodrigues, J.M.F. (2017b). Framework for a Hospitality Big Data Warehouse: The Implementation of an Efficient Hospitality Business Intelligence System. International Journal of Information Systems in the Service Sector, 9 (2), 27-45. Doi: 10.4018/IJISSS.2017040102
Rodrigues, J.M.F., Ramos, C., Pereira, J., Sardo, J., & Cardoso, P.J.S. (2019). Mobile Five Senses Augmented Reality System: technology acceptance study. IEEE ACCESS, 7(1), 163022-163033. (Print ISSN: 2169-3536; Online ISSN: 2169-3536). Doi: 10.1109/ACCESS.2019.2953003
Rodrigues, J.M.F., Lessa, J., Gregório, M., Ramos, C., & Cardoso, P.J.S. (2016). An Initial Framework for a Museum Application for Senior Citizens. In Proc. of the 7th International Conference on Software Development and Technologies for Enhancing Accessibility and Fighting Info-exclusion (DSAI 2016). ACM, New York, NY, USA, 414-421. Doi: 10.1145/3019943.3020002
Sharma, G.K., & Sharma, P. (2016). A study on data mining algorithms for tourism industry. International Journal of Latest Trends in Engineering and Technology, 7(1), 580-587.
Sheldon, P.J. (1989). Travel Industry Information Systems in Witt, S. & Moutinho, L. (ed) Tourism Marketing and Management Handbook (pp. 589-592). Prentice Hall, London.